Stunning Pretty Scale Designs & More!
What constitutes a visually appealing and easily digestible representation of numerical data? A well-chosen method for presenting data can dramatically improve understanding and impact.
A "pretty scale" in data visualization refers to a method of scaling numerical data for visual presentation that optimizes clarity, aesthetics, and comprehension. This involves selecting an appropriate range and interval for the data representation, often incorporating visual cues like color gradients, logarithmic axes, or custom tick marks, depending on the specific context and desired message. For example, visualizing sales figures across different product categories might benefit from a scale that highlights the relative differences between categories rather than focusing on the exact numerical values. An effectively chosen scale, therefore, can transform complex data into a clear and insightful visual narrative.
The importance of a well-designed data scale lies in its ability to improve data understanding and communication. Effective visualizations enhance comprehension, reducing the cognitive load on the viewer and accelerating insights. This is especially crucial in fields like scientific research, business intelligence, and public policy, where presenting data concisely and persuasively is vital. A poorly chosen scale can distort the message, leading to inaccurate interpretations or even misleading conclusions. The careful selection of a "pretty scale" is therefore critical to avoid misinterpretations and promote accurate data-driven decision-making. Effective visual representations not only support understanding but also drive action.
Moving forward, we will delve into specific examples of effective data scaling strategies and explore best practices for creating visually compelling and insightful data representations across various fields, emphasizing how optimal scaling choices enhance communication and understanding.
Pretty Scale
Effective data visualization hinges on the careful selection of scales. A well-chosen "pretty scale" enhances clarity, facilitates comprehension, and minimizes misinterpretation. The following key aspects are crucial for constructing such scales.
- Visual appeal
- Data relevance
- Clear communication
- Accurate representation
- Appropriate range
- Logical intervals
- Contextual awareness
- User engagement
Visual appeal, for instance, involves utilizing aesthetically pleasing color schemes and formatting. Data relevance ensures the scale accurately reflects the underlying data. Clear communication emphasizes the scale's ability to convey insights readily. Accurate representation avoids distortions, allowing for reliable conclusions. Appropriate range ensures the scale encompasses the full data spread; logical intervals make the data easy to read and interpret. Contextual awareness necessitates the scale's alignment with the overall message. User engagement considers how viewers will interact with the scale, potentially leading to increased comprehension and insights. These key aspects, when considered in combination, yield a "pretty scale," enabling accurate insights and clear, impactful data presentations.
1. Visual Appeal
Visual appeal in data presentation is intrinsically linked to the effectiveness of a "pretty scale." An aesthetically pleasing and well-designed visualization significantly impacts comprehension. A data scale, even with accurate numerical representation, loses its potential if the visual presentation is uninviting or confusing. A strong visual foundation enhances the likelihood of data insights being accurately perceived and effectively communicated.
- Color and Contrast
Effective use of color and contrast is critical. High contrast between data points and the background ensures readability, especially for individuals with visual impairments. Color palettes should be chosen thoughtfully, avoiding jarring or overly saturated hues. Consistent color usage across different elements of the visualization contributes to clarity and cohesion. Examples include using distinct colors for different categories or employing a gradient to show a range of values. Inaccurate or inappropriate color choices can distort perception, rendering the data scale less effective.
- Typography and Formatting
Clear and legible typography is essential. Appropriate font choices, sizes, and spacing enhance readability. Consistent formatting across different elements reinforces visual clarity and enhances the overall aesthetic appeal. Examples include using consistent font sizes for axis labels, titles, and data points. Poorly chosen fonts or formatting choices can disrupt visual flow, creating cognitive obstacles for viewers trying to interpret the data.
- Layout and Organization
A well-organized layout enhances the overall visual appeal and aids in understanding. The arrangement of elements, such as data points, axis labels, and titles, should enhance, rather than obscure, the data's message. Cluttered or disorganized layouts lead to a disjointed understanding of the data. Examples include utilizing whitespace effectively, arranging charts logically, and prioritizing the most important data elements through placement and size. Proper alignment, positioning, and visual hierarchy all contribute to a more engaging and comprehensible visual representation of the data.
- Use of Visual Cues
Adding visual cues, such as shapes, icons, or patterns, can further enhance understanding and engagement. These visual cues should be employed strategically, with clear and concise descriptions. Overuse of visual cues can lead to confusion and detracts from the data's core message. Examples include employing different shapes for different categories, using a line graph to visualize trends, or highlighting key data points through distinct visual markers. Choosing the correct visual cues for the task is critical.
Ultimately, visual appeal within a "pretty scale" is not an aesthetic exercise alone; it's a critical element for effectively communicating data-driven insights. The choice of colors, fonts, layout, and other visual elements directly impacts the viewer's ability to quickly grasp and retain insights. A visually engaging and comprehensible presentation is more likely to lead to meaningful conclusions.
2. Data Relevance
Data relevance is fundamental to a "pretty scale." A scale's effectiveness hinges on its alignment with the data it represents. Irrelevant data, presented in a visually appealing format, remains ultimately meaningless. A "pretty scale" must accurately reflect the characteristics of the data set it's visualizing, ensuring the visual representation correctly conveys the underlying message. The scale should not distort or misrepresent the data's essential features, thereby undermining its value and potential for effective communication. If the data itself is skewed, incomplete, or poorly defined, no amount of visual artistry can salvage the scale's inherent limitations. A well-designed scale that misrepresents flawed data is not a "pretty scale" in the true sense. The data's meaning and context should be paramount.
Consider a financial report depicting quarterly sales figures. If the scale inappropriately emphasizes minor fluctuations, overshadowing significant trends, or includes extraneous data points, the data's value diminishes. An effective visualization highlights key trends; otherwise, the "pretty scale" becomes irrelevant, potentially masking important insights. In epidemiological studies, a "pretty scale" must correctly represent disease incidence rates, providing context for the interpretation of data. An inappropriate scaling choice might result in an underestimation of the impact of a particular trend. An accurate portrayal of the data is crucial in guiding evidence-based policy decisions and public health initiatives. Similarly, in scientific research, the scale must correctly represent the relationship between variables for meaningful results.
Understanding the connection between data relevance and a "pretty scale" is vital in achieving meaningful conclusions. A scale's aesthetic appeal is secondary to its accuracy in reflecting the data's core message. Inaccurate or irrelevant data rendered visually appealing remains problematic; a "pretty scale" ultimately serves to enhance understanding of relevant data, enabling informed decisions based on valid interpretations. Without relevance, even the most visually engaging scale fails its core purpose of effectively conveying insights. The strength of a "pretty scale" is determined by its ability to faithfully present the information relevant to the specific goal of the visualization.
3. Clear Communication
Clear communication is paramount in data visualization. A "pretty scale," while visually engaging, is ultimately ineffective if the data it presents cannot be easily understood. Effective communication hinges on the ability of the visualization to convey information accurately and unambiguously to the intended audience. The design choices comprising a "pretty scale" must, therefore, support, not hinder, clarity.
- Accessibility and Readability
A key facet of clear communication is accessibility. The scale should be designed in a way that accommodates a diverse range of viewers. This includes considering visual limitations such as color blindness or low vision. Font sizes, color contrasts, and symbol choices need careful consideration to ensure readability and comprehension for everyone. Effective examples include using clear, concise labels for axes, legends, and data points, avoiding overly complex or visually overwhelming designs. A clear, accessible presentation ensures the intended message reaches all viewers, enhancing comprehension and fostering a greater impact. Conversely, confusing or poorly designed scales create barriers to understanding for many viewers.
- Contextual Understanding
The scale must accurately reflect the context within which the data is presented. Incorporating context into the visualization helps viewers to grasp the significance of the data and its implications. This involves clearly labeling units, providing appropriate units of measurement, and making relevant background information easily accessible. A visualization lacking context may leave viewers puzzled or struggling to interpret the data's meaning. Clear communication establishes the scale within its appropriate setting. Examples include clearly defining the population sample for a survey result or the timeframe for a trend analysis in a business report. The absence of contextual information can distort the meaning and limit the usefulness of the presented data.
- Effective Visual Hierarchy
A clear visual hierarchy facilitates comprehension. The design should prioritize and highlight the most important data points. This might be achieved through variations in size, color, or placement. Visual cues, such as data highlighting and emphasis through bolding or contrasting color, are fundamental to emphasizing crucial data. Examples of visual hierarchy include using larger font sizes for key statistics or utilizing color intensity to highlight outliers. In contrast, a cluttered design that lacks clear distinctions between data elements hinders interpretation, thus obfuscating the core message of the visualization.
- Unambiguous Data Representation
The scale must present data without ambiguity. Each elementdata points, axes, legendsshould communicate a single, unambiguous message. Data points should not be visually confusing or misleading. The chosen method of representing databar graphs, line charts, or other typesmust be appropriate and unambiguous. Examples include utilizing appropriate chart types (e.g., line graphs to depict trends, bar charts to compare categories) and ensuring clear annotations of all labels and elements. Misrepresenting or overly abstract representations of data erode the communication goal, reducing the scale's utility and clarity.
These facets of clear communication are inseparable from a "pretty scale." Effective communicationaided by clear, accessible visualizationsis crucial for understanding, interpreting, and acting upon presented data. A well-designed scale, therefore, balances aesthetics with clarity and provides accessible and accurate insights to the audience. The goal is to make the data tell its story effectively.
4. Accurate Representation
Accurate representation is fundamental to the effectiveness of a "pretty scale." A visually appealing visualization, devoid of accurate data representation, is ultimately misleading and useless. The scale's design must mirror the underlying data's characteristics truthfully, preventing misinterpretation and fostering trust in the presented information. This facet underscores the critical link between the visual presentation and the substance of the data itself.
- Appropriate Scaling and Units
Accurate representation begins with employing an appropriate scale. A scale must encompass the full range of data values, avoiding truncation that distorts the visual representation. Units of measurement must be clearly and consistently labeled, eliminating ambiguity about the data's magnitude. Inconsistent units or inappropriate scaling methods can lead to significant misinterpretations. For example, graphing population growth over several decades requires a scale that effectively displays long-term trends, avoiding compression that obscures the true rate of growth. Similarly, financial reports must use consistent units for currency and time intervals. Failing to adhere to these fundamental principles compromises the accuracy and ultimately the value of the visualization.
- Correct Data Representation Types
The choice of visualization method must accurately reflect the nature of the data. A bar chart may be suitable for comparing categories, while a line graph is best for illustrating trends over time. Mismatched visualization and data characteristics create inaccuracies. For instance, using a pie chart to demonstrate a trend over several years is inappropriate and would misrepresent the data. Appropriate use of charts and graphs directly corresponds to accurate data representation and enhances the understanding of presented data.
- Minimizing Distortion
Visualizations must avoid distortions that misrepresent the data's true relationship or distribution. For example, logarithmic scales are useful for displaying data with a wide range of values, but using a linear scale for such data can significantly misrepresent the underlying trends. The selection of appropriate tools, like logarithmic scales or other specific graph types, is crucial in minimizing distortion. Likewise, the placement of data points and the use of color gradients should not exaggerate or diminish differences between data points, preserving the data's integrity within the visualization.
- Avoiding Biases and Errors
Careful attention to data collection and input errors is essential. Incorrect data points, if incorporated into the visualization, inevitably compromise the representation's accuracy. The visualization itself must not introduce any bias that affects the presentation of the data. For instance, using colors that evoke specific emotions or subtly emphasize certain data points can introduce an unintended bias that distorts the presentation. Identifying and correcting errors before creating a "pretty scale" is imperative to prevent misleading interpretations. Accurate data entry and a rigorous review process in the initial stages are crucial to achieving accurate representation.
In summary, accurate representation is the bedrock upon which a "pretty scale" is built. A visually compelling visualization is worthless if its foundation is inaccurate data. Attention to detail in scaling, representation types, distortion avoidance, and bias elimination guarantees a data-driven, truthful presentation. A well-designed visualization should always be consistent with the data, enabling reliable conclusions and minimizing potential misinterpretations. Ultimately, the scale's "prettiness" must support, not obscure, its primary function of accurately reflecting and communicating the data's essence.
5. Appropriate Range
The appropriate range of a scale in data visualization is crucial to the effectiveness of a "pretty scale." A properly selected range ensures that the data's essential features are accurately represented, avoiding misinterpretation. A poorly chosen range can obscure key trends, exaggerate minor fluctuations, or even mislead the viewer about the data's overall significance. The selection of the appropriate range is inextricably linked to the clarity and accuracy of the visual presentation.
Consider a dataset illustrating the average daily temperature in a city over a year. If the scale starts at 0C and ends at 30C, subtle variations within a range of 10-25C may be overlooked, while the entire range of 0-30C might not provide a meaningful overview of the patterns or seasonal changes. However, if the scale is focused on the range of 15C to 25C, the visual will effectively highlight the daily variations and the seasonal shift more clearly. Similarly, analyzing stock prices across five years necessitates a range that captures the full fluctuation, perhaps starting from a significant low point and extending through major highs, highlighting the long-term trend. The choice of range directly impacts the visibility of key insights. In the case of a company's sales data, a range that captures the peak sales period clearly allows viewers to understand the seasonality and sales cycles and allows accurate comparisons between periods. Conversely, a range that excludes the highest sales period will obscure important performance indicators.
Proper range selection in data visualization directly affects the viewer's ability to interpret the data accurately. A well-defined range enhances the clarity of the visual representation, leading to a more effective and accurate understanding of the data's inherent characteristics. Effective range selection contributes directly to the overall quality of the visualization. By focusing on the relevant range, the visualization effectively highlights the key patterns, trends, or outliers present within the data, enabling informed decision-making. Conversely, an inappropriately chosen range can lead to significant misinterpretations and render the visualization useless. Careful consideration of the range is essential for producing insightful and effective "pretty scales." By carefully considering the range, the visualization better focuses on the information critical to the audience's needs. This clarity is vital for effective communication, empowering better decision-making, whether in scientific research, business analysis, or other domains.
6. Logical Intervals
Logical intervals in data visualization are integral components of a "pretty scale." A well-structured scale, utilizing logical intervals, fosters comprehension and avoids misinterpretations. This aspect ensures the visual representation accurately reflects the underlying data's characteristics and enhances the overall effectiveness of the visualization. Meaningful insights are more readily gleaned when intervals are not arbitrary, but rather structured in a way that intuitively reflects the data's pattern.
- Consistency and Readability
Consistent intervals are critical for readability. Visualizing data with intervals that have no logical relationship hinders understanding. This lack of order makes it difficult to identify trends and patterns. Conversely, logical intervals, such as increments of 10, 100, or 1000, contribute to quick and clear data interpretation. Data points are more easily compared across categories when intervals remain consistent. Consider a graph displaying sales figures; if the intervals are irregular or inconsistent, the viewer struggles to make accurate comparisons between different periods. Consistent intervals, in contrast, highlight trends and enable efficient analysis.
- Accurate Representation of Data Distribution
Logical intervals help accurately reflect the data's distribution. They provide a framework for understanding the data's density and concentration within specific ranges. For example, a dataset showing population density across counties necessitates intervals that align with the range of densities observed. If intervals are too large, the visualization may mask subtle variations in density. In contrast, appropriately chosen, logical intervals reveal variations in population density precisely and accurately. The representation is accurate and not misleading. This is critical for understanding patterns or outliers that may be hidden due to an inappropriate interval selection. A graph depicting stock prices across several years requires intervals that reflect the relevant fluctuations, not arbitrarily spaced intervals that obscure patterns.
- Avoiding Misleading Visualizations
Logical intervals minimize the risk of misleading visualizations. Inaccurate intervals can distort perceptions of relationships between variables. For example, an improper selection of intervals for a graph depicting temperature fluctuations across seasons can exaggerate minor fluctuations, or obscure important trends in temperature changes. Logical intervals, however, accurately represent the data's characteristics, preventing misleading insights or incorrect conclusions. The visual representation remains faithful to the data.
- Facilitating Accurate Comparisons
Logical intervals are fundamental to accurate comparisons. When intervals are carefully chosen, comparing different categories or periods becomes more straightforward. For instance, comparing sales figures across different product categories on a chart becomes easier with logical, consistent intervals. By ensuring the intervals are relevant and consistent, the visual comparison accurately reflects the differences between the categories. This is essential in facilitating comparisons and deriving meaningful insights.
Ultimately, logical intervals are a cornerstone of an effective "pretty scale." By ensuring intervals are consistent, reflective of data distribution, and minimize distortions, the visualization becomes a powerful tool for accurate data interpretation and effective communication of insights. Such a visualization accurately reflects the relationships within the data.
7. Contextual Awareness
Contextual awareness in data visualization, especially within the framework of a "pretty scale," is not merely an aesthetic consideration; it is fundamental to accurate interpretation and effective communication. The context surrounding the data profoundly shapes how it should be presented and understood. A visualization stripped of its context risks misrepresentation, leading to flawed conclusions. Understanding the specific situation, the intended audience, and the goals of the presentation is crucial for creating a scale that effectively communicates the data's meaning.
- Audience Considerations
The intended audience directly influences the design and interpretation of a "pretty scale." A visualization intended for specialists in a particular field will differ significantly from one targeting a broader, non-expert audience. Technical jargon, complex visualizations, and intricate details might be suitable for the former but overwhelming for the latter. A clear understanding of the intended audience's background and knowledge level dictates the level of detail and complexity appropriate for the visualization. A "pretty scale" tailored to the audience maximizes comprehension and minimizes confusion.
- Data Source and Methodology
The origin and methodology of the data greatly influence the appropriate scale's design. Knowing the data collection methods, potential biases, and limitations is vital for selecting a scale that accurately represents the underlying information. The source of data, like a survey or an experimental study, significantly influences the interpretations derived from the visualization. A "pretty scale" incorporating these aspects allows viewers to grasp the limitations and potential biases embedded in the dataset.
- Specific Objectives and Purpose
The purpose and specific objectives behind the visualization guide the creation of a meaningful "pretty scale." A scale designed to highlight trends will differ from one focused on comparing specific categories or identifying outliers. For instance, a visualization to demonstrate the effectiveness of a new marketing strategy will focus on key metrics relevant to that strategy. A "pretty scale" aligns with the visualization's objective, ensuring that the presented data meaningfully supports the intended message.
- Impact and Implications of the Findings
Considering the implications and potential impact of the data's findings within the overall context is vital for creating a "pretty scale." The scale should effectively convey the significance of the data, whether it highlights a successful trend, reveals an urgent issue, or demonstrates a potential risk. The context within which the findings apply should be clearly presented. A "pretty scale," aware of these implications, ensures data's impact is not lost in visual complexity.
Contextual awareness, therefore, is more than just a consideration; it's a crucial element of data visualization's effectiveness. By deeply understanding the circumstances surrounding the data, the intended audience, and the desired outcomes, the creation of a "pretty scale" is not merely about aesthetics but rather about effectively communicating critical information within its specific context. A contextually aware scale ensures the insights derived from data are accurate, meaningful, and ultimately useful in informing decisions within that context.
8. User Engagement
User engagement in data visualization is not merely a desirable trait; it's a critical component of a successful "pretty scale." A compelling visualization actively draws the user into the data, fostering comprehension and retention of key insights. A well-designed scale, thus, actively encourages user participation, motivating them to explore, understand, and interact with the information presented. The effectiveness of a visualization is intrinsically tied to user engagement.
- Intuitive Navigation and Interaction
A user-friendly interface is crucial. Navigation should be intuitive, enabling users to easily explore different facets of the data. Interactive elements, such as clickable charts or customizable filtering options, further enhance user control and engagement. Example visualizations that successfully incorporate these principles will smoothly allow viewers to navigate different aspects, drill down into details, and dynamically adjust perspectives without encountering complexities in the presentation. Conversely, a confusing or unresponsive visualization can quickly disengage users, reducing the impact and effectiveness of the presented information.
- Visual Clarity and Accessibility
Visual clarity is fundamental. A clear and concise visualization allows users to readily identify patterns, trends, and outliers. A well-structured layout, readily understandable color schemes, and easily readable fonts enhance comprehension and engagement. Example visualizations effectively use a balanced and non-distracting color palette, employing clear visual cues for key data points, and avoiding overcrowding. Conversely, a visually overwhelming or confusing visualization becomes a barrier to engagement and comprehension. Inclusive design principles, such as considering users with visual impairments or cognitive differences, contribute to broad engagement.
- Informative and Actionable Data
Meaningful insights are necessary. The data presented should be relevant and actionable. Users need to see a clear connection between the visualization and their needs or goals. Example visualizations effectively showcase data that directly addresses a specific question, enables the user to draw conclusions, and empowers data-driven decisions. Conversely, presenting irrelevant data or lacking context in the visualization diminishes user engagement and motivation, as users perceive little benefit to the presented data.
- Feedback and Exploration
User feedback and exploration are crucial. Visualizations should encourage exploration. Tools enabling interactive exploration, data filtering, and zooming can foster deeper engagement. Example visualizations allow users to investigate data at varying levels of detail and manipulate elements to understand complex relationships. Conversely, a static visualization without the ability to explore or react discourages active engagement and leads to a passive consumption of information, potentially limiting comprehension and insights.
Ultimately, a "pretty scale" that is user-focused and interactive encourages active involvement with the data. Visual clarity, intuitive navigation, and the ability to derive practical insights contribute directly to higher levels of user engagement, resulting in enhanced comprehension, meaningful insights, and successful communication of the data's message. Such engagement is critical in achieving the intended purpose of presenting and interpreting data.
Frequently Asked Questions
This section addresses common inquiries regarding "pretty scales" in data visualization. These scales aim to present numerical data in a clear, compelling, and easily understandable manner. Properly constructed scales enhance the communication of insights and facilitate informed decision-making.
Question 1: What distinguishes a "pretty scale" from other methods of data scaling?
A "pretty scale" prioritizes visual appeal and ease of understanding alongside accurate representation. While other scaling methods may focus solely on mathematical precision, a "pretty scale" balances visual clarity with accurate data reflection. Key characteristics include visually appealing aesthetics, logical intervals, and effective communication of the underlying data's story. This method aims to enhance the overall impact and comprehension of data presented, making it intuitive for a broader audience.
Question 2: How does the selection of a color scheme impact the effectiveness of a "pretty scale"?
Color choice significantly impacts comprehension. A well-considered color scheme enhances readability and engagement. Effective choices are high-contrast, avoiding confusion and accommodating potential visual impairments. Selecting a color scheme that complements the data's message and resonates with the intended audience enhances the overall impact and understanding. Conversely, inappropriate or poorly executed color choices can detract from the clarity and effectiveness of the visualization.
Question 3: What role do logical intervals play in creating a clear and effective "pretty scale"?
Logical intervals are critical in a "pretty scale" because they ensure consistent and accurate data representation. Intervals that are too large or too small can misrepresent patterns and trends within the data, while logical intervals facilitate accurate comparisons across categories and periods. Employing appropriate spacing for increments directly contributes to the overall clarity and accuracy of the presentation, allowing for more precise interpretation.
Question 4: Are there specific considerations when creating a "pretty scale" for diverse audiences?
Diverse audiences require adaptable design considerations. Understanding the knowledge level and background of the target audience is crucial. Complex visualizations may overwhelm less-specialized audiences. Clear labeling, appropriate terminology, and a balance between visual appeal and clarity are essential for effective communication with diverse audiences. Accessibility features, such as sufficient color contrast, should be incorporated to ensure inclusivity.
Question 5: How does a "pretty scale" differ from a misleading visualization?
A "pretty scale" accurately reflects the underlying data. Misleading visualizations, in contrast, use visual representations that distort or obscure data characteristics. This distortion can lead to misinterpretations and flawed conclusions. A "pretty scale" strives for accuracy and transparency. Conversely, misleading visualizations use design choices to exaggerate or minimize differences, resulting in an inaccurate or deceptive presentation of data.
These answers highlight critical aspects of constructing an effective "pretty scale." By carefully considering visual appeal, logical intervals, contextual awareness, and engagement, data can be effectively presented and interpreted.
Moving forward, specific examples of well-designed and poorly designed scales in data visualization will be explored in detail.
Conclusion
This exploration of "pretty scale" in data visualization underscores the critical role of effective visual communication in conveying insights from numerical data. The analysis highlights that a well-designed scale goes beyond mere aesthetics; it directly impacts understanding and interpretation. Key factors contributing to an effective "pretty scale" include visual appeal, data relevance, clear communication, accurate representation, appropriate range, logical intervals, contextual awareness, and user engagement. Each element, when considered holistically, results in a visualization that accurately reflects the underlying data while fostering comprehension and actionable insights. The importance of avoiding misleading representations, which can result from inappropriate scaling or poorly considered visual design choices, cannot be overstated. A "pretty scale" is not simply a decorative element; it is an essential tool for deriving accurate conclusions from data.
The creation of effective data visualizations requires a careful consideration of the interplay between visual presentation and the underlying data. A "pretty scale" serves not only to make data more appealing but crucially to enhance its accessibility, clarity, and understanding. As data continues to grow exponentially in volume and complexity, the need for effective visualization techniques, including "pretty scales," becomes increasingly paramount. Data-driven decision-making hinges on the ability to interpret information accurately and efficiently. The continued development and application of effective visual communication strategies, informed by principles of a "pretty scale," are therefore vital for progress across diverse fields, from scientific research to business analytics and public policy.
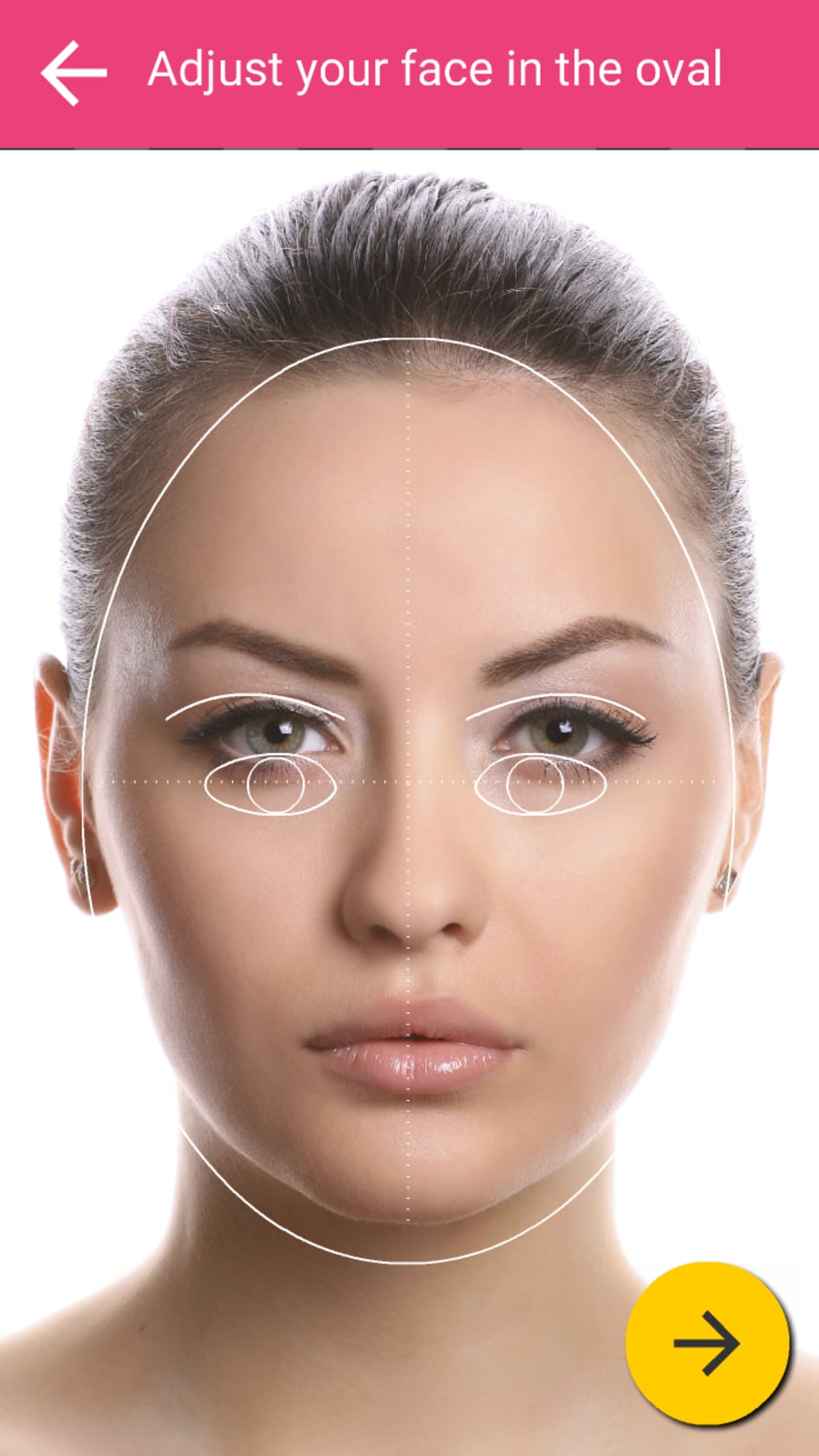
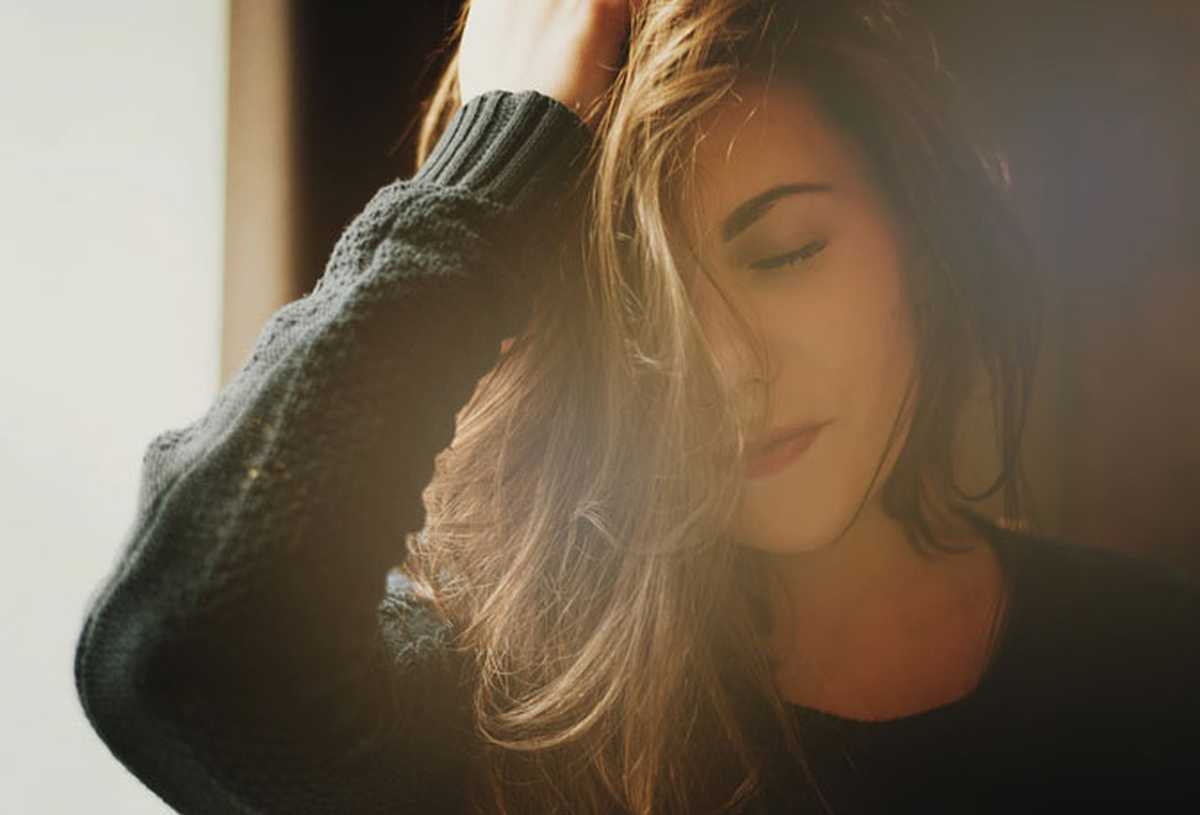
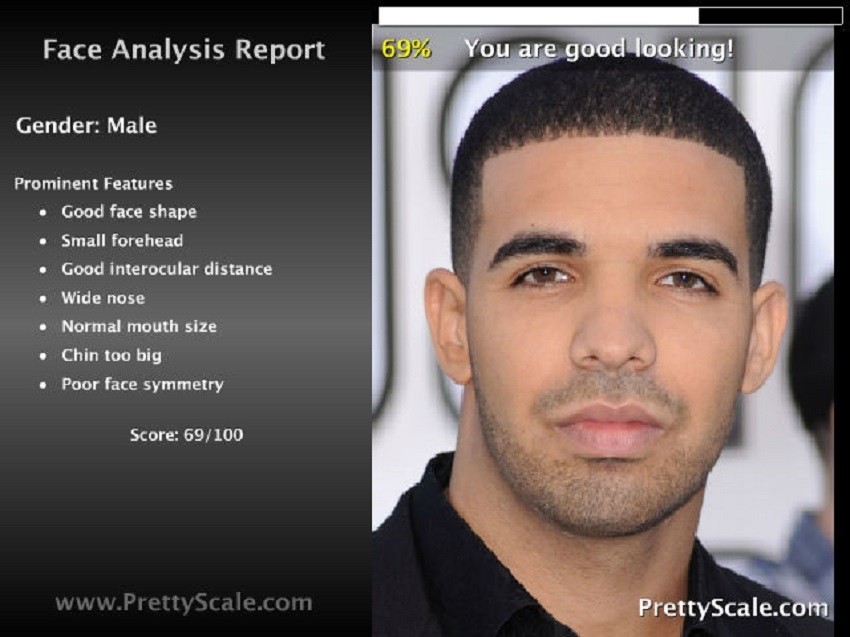